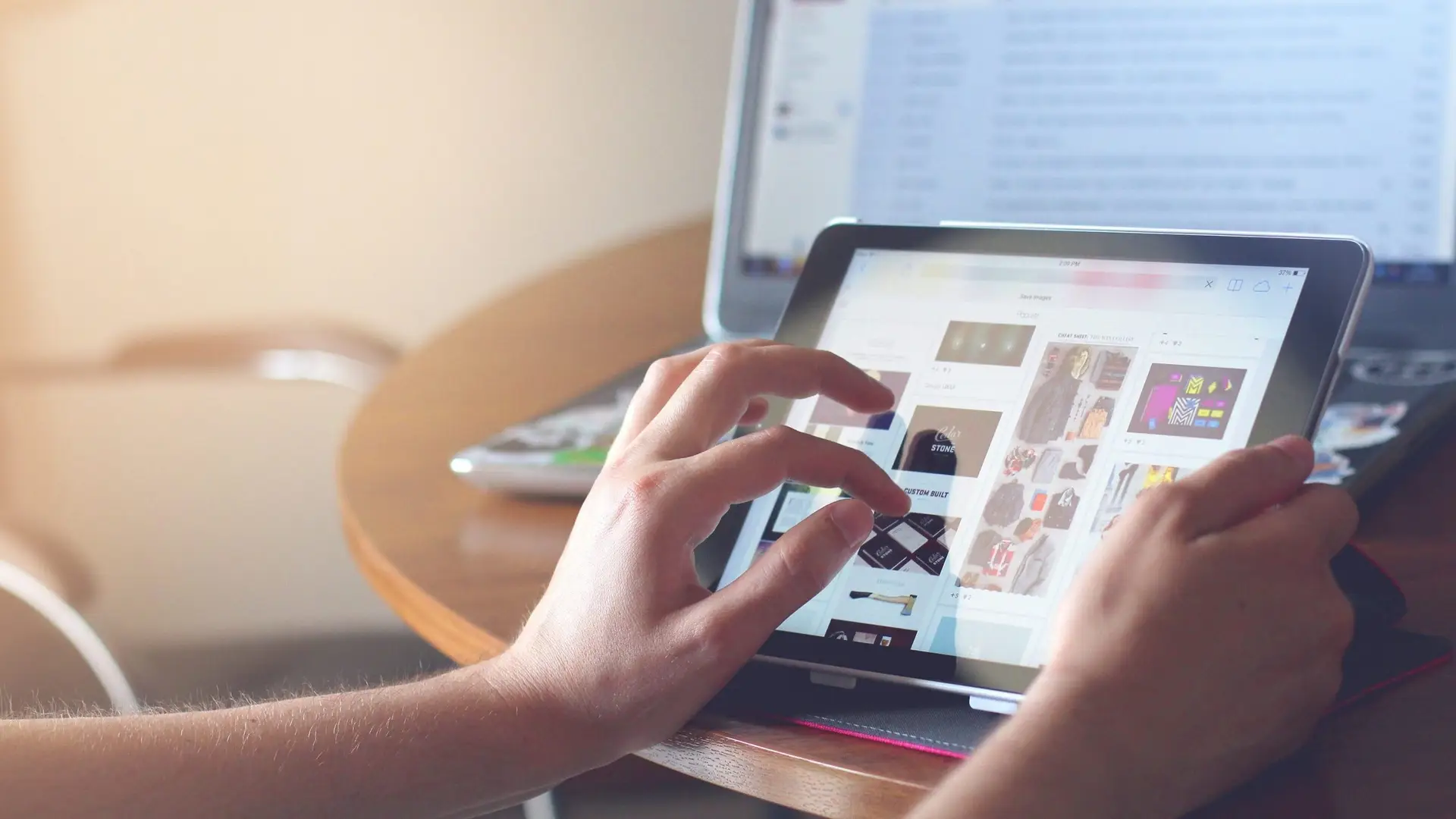
Data labelling – Outsourcing Guide
An overview
Industries that practice machine learning (ML) and artificial intelligence (AI) from self-driving cars, industrial automation, retail, agriculture and many more – look for labelled data to train their ML algorithms to identify trends and make predictions. Data annotation requires a specialist team for the labelling of training datasets with multiple formats such as texts, images, graphics, videos etc. Plus a sizeable amount of data needs to customised to create an AI or ML based model – as greater the datasets, greater the accuracy.
In this situation, your business will call for quick and efficient data labelling to get your product rolled out. Does that mean you should outsource your data labelling team?
In-house or Outsourcing?
An internal data labelling team would provide direct control over the data labelling process and data security. While an in-house team is beneficial for these reasons – this comes at a cost, which includes the enormous time and resources required to find and train a qualified group, provide a safe workspace and the appropriate tools; in addition the managerial costs that go with this. If the workload fluctuates seasonally or from project to project, that makes things a bit more complicated for the HR & Training teams.
Outsourcing data labelling turns the table on the in-house team. It is time and cost-sensitive and is appropriate for both high-volume data for long-term projects or short projects that require quick turnaround; leaving you and your team to focus on the core model & algorithms. And here are a few reasons if you chose to outsource:
Benefits of outsourcing:
- Quality Datasets
The quality and accuracy define the success of the AI or ML model right from the accuracy of the points placed on the digital content. The best accurate output is only attainable with experts devoted to AI or ML jobs. Our data quality and accuracy are measured using human and automated QA techniques, including consensus algorithm, benchmarking and gold standard, Cronbach’s alpha test or a combination of these.
- Eliminate bias
The most common bias is when you use data to train your model that does not accurately represent the environment that the model operates in real-life use. Under such circumstances, data accuracy gets compromised and impacts the productivity of ML models. Biased results from training data are also influenced by factors like cultural or other stereotypes of the team. When our remote team works in tandem with your team we can ensure that this doesn’t hinder the process.
- Faster turnaround
Training the machines requires a sizable number of labelled datasets to ensure that the model receives most of the variation learnt from the data and produces accurate results. Additionally, if the project relies on deep learning, massive data is required to train the model to comprehend the intricacies of the algorithms and deliver accurate results. In such a case, our in-house team can help you scale faster for a quick turnaround.
- Data Confidentiality
Due to data protection regulations, such as those involving PII or PHI, or other factors, few businesses prefer to keep data annotation in-house. However with a good Non Confidentiality Agreement in place – keeps vendors from divulging sensitive information. Syntax global takes privacy and protection of data seriously with all safeguards in place – read more about this on our Data protection and Privacy page here. https://syntaxglobal.com/privacypolicy
Summing up:
The accuracy of the annotation will affect how well the algorithm works. Evaluate golden trio of Quality, Speed and Cost when choosing the right vendor. Syntax Global can help with the quick competitive edge and enabling you to expand smoothly.